What is Industry 4.0?
Industry 4.0 is the integration of many emerging technologies that collect and leverage Industrial Big Data to drive manufacturing and supply chain automation, provide real-time insights, and close communication feedback loops for faster decision-making in the manufacturing process.
Video: Industry 4.0 explained
The next stage of factory automation is only possible if an organization’s cyber-physical systems create and collect high-fidelity data, automatically analyze it, and pass relevant information to the right machine or person, at the right time. In addition, the data must be in a usable format for the recipient, whether human or machine.
Smart machines collect and analyze data to direct other devices and people. The data is connected across all parts of an organization to increase manufacturing productivity in real-time. With this level of big data connectivity and intelligence, factories, plants, warehouses, and machines can be fully automated.
Not all necessary data comes from machines. Human data entry from engineering, procurement, logistics, and customer service must be accurate and high-quality. Humans are often responsible for entering foundational or source information like CAD data, pricing, and customer feedback. Inaccuracies within the data input can lead to costly errors. These errors must be mitigated for the successful implementation of Industry 4.0.
Industry 4.0 Glossary of Terms
What is Industry 4.0 Infographic

Share the Industry 4.0 Infographic On Your Site
The History of Industrial Revolutions
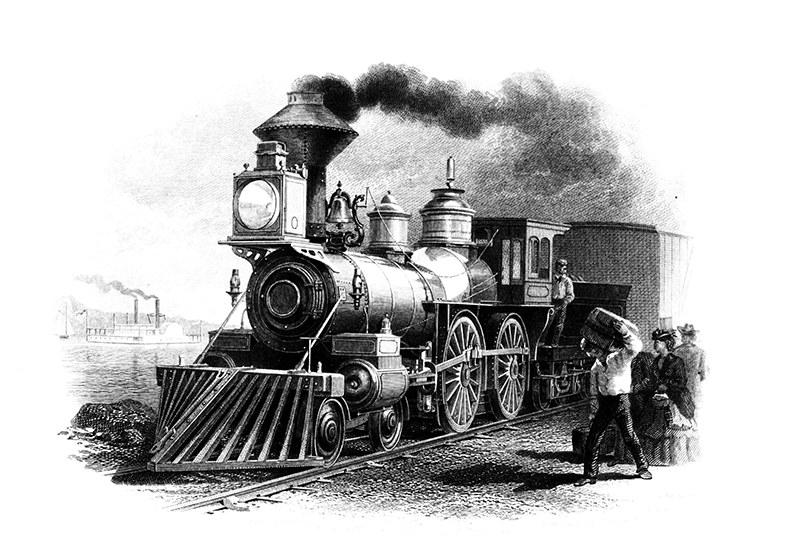
The First Industrial Revolution, Mechanization 1760-1830
Manufacturing was primitive before 1760 and limited to human and animal power. Some sophisticated systems existed, but manufacturing capacity was limited. The Industrial Revolution changed everything.
Characterized by mechanization, The First Industrial Revolution introduced machines to the manufacturing process. Manufacturing processes began harnessing steam and water to power machines instead of relying solely on human and animal strength. Iron and steel factory machines became prominent, and the internal combustion engine made its way to the factory floor.
The Second Industrial Revolution, Mass Production 1870-1914
The assembly line, process standardization, and electric power machines led to mass production. Railways moved raw materials and finished products faster than wagons and horses. And the flow of information through the telegraph, and eventually, the telephone all contributed to another manufacturing renaissance.
Henry Ford was a prominent figure in the Second Industrial Revolution. He introduced the first assembly line for a complete automobile. The assembly line cut production time from over 12 hours to one hour and 33 minutes for Ford’s Model T!
The Second Industrial Revolution opened new supply chains, led to mass production, and made products more readily available to the average person.
The Third Industrial Revolution, Digitalization 1950-2002
In the 1950s, computers became a staple of manufacturing. Advances in telecommunication, electronics, and IT infrastructure lead to widespread automation using robots and PLCs (programmable Logic Controllers). Efficient machines paired with computers performed tasks that only humans were capable of in the past.
The Fourth Industrial Revolution (Industry 4.0), Interconnectivity 2011-present
Deep system integrations that pass large-scale data between machines became possible by advances in data storage, networking, and artificial intelligence. Companies can now equip components within a manufacturing facility with sensors that enable machinery to assist with complex tasks more comprehensively. These sensors pass data back to central systems for distribution throughout the enterprise, providing diagnostics and analysis to streamline processes. The foundation of Industry 4.0 is the interconnectivity of systems, paired with real-time data and machine learning that drive factory automation.
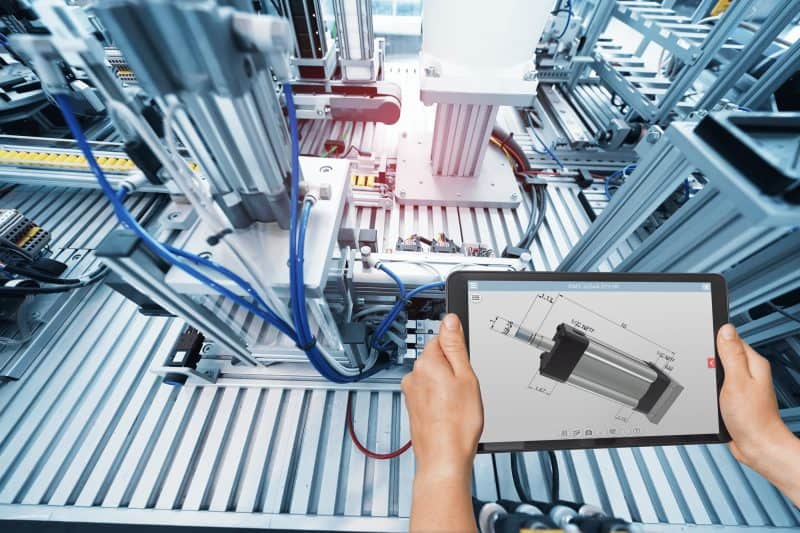
Requirements of Industry 4.0
The Fourth Industrial Revolution is all about data, communication, and interconnectivity.
The goal of Industry 4.0 is to automate manufacturing through cyber-physical systems that collect, analyze, and communicate data between interconnected networks.
We will cover four requirements for achieving the automated data intelligence needed to achieve Industry 4.0.
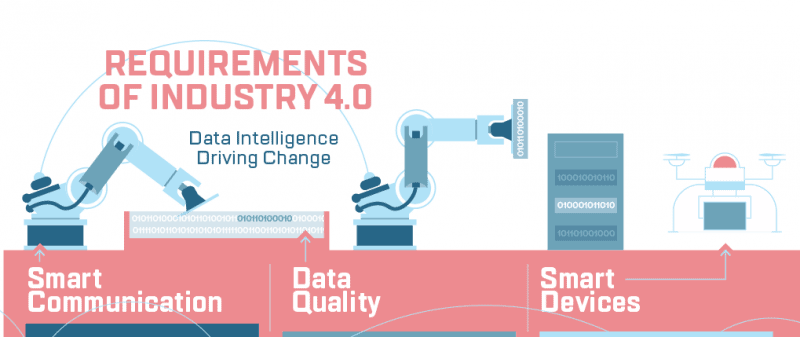
Smart Communication
Communication has advanced significantly over the last 200 years.
In America, mail systems like The Pony Express transported physical letters through a network of horses relaying mail across the country. The Pony Express was a lot faster than the old methods where letters slogged on wagon trains for weeks or months.
The invention of the telegraph turned the world upside down, bringing instant communication across hundreds of miles. Locomotives started carrying large amounts of information and documents by railway around the same time.
After the invention of the telephone, people were able to have conversations over long distances. Then came computer intranets. And finally, the expansion of connected intranets to the World Wide Web and the internet we use today.
Ever since the 1990s, the pace of data communication has increased exponentially.
Smart communication drives Industry 4.0
Industry 4.0 is a communication and interconnectivity revolution. New technologies are finally making smart communication possible. Instead of humans communicating and analyzing data with some help from machines, smart devices are now communicating with some assistance from humans.
The overwhelming amount of data communication is now too vast for humans alone to analyze and make decisions. Additionally, the quantity of data, along with advancements in machine learning and artificial intelligence, have enabled machines to speed up manufacturing by processing data at a pace and scale never seen before.
Finally, machines and systems are beginning to bypass former human bottlenecks by communicating and making necessary decisions without human involvement.
The goal of Industry 4.0 is smart communication: Machines communicating with other devices leading to factory automation.
Data Quality
Data is the cornerstone of Industry 4.0. Factory automation is dependent on the collection and communication of data. However, data intelligence and communication are only as good as the quality of the data. If one portion of data is incomplete, the whole system can fail.
The conversation about data collections needs to shift. For too long, collecting as much data as possible has been championed over gathering useful data that translates between systems. Actionable insights and automated decision-making by machines and computer systems require focus over the next decade to achieve Industry 4.0. In other words, the quality of data is more important than the quantity of data. A lot of low-quality data means more resources being consumed for less accurate outputs.
Smart Devices
An example of Industry 4.0 connected smart devices is visual sensors attached to robotic arms that send and receive data. It also includes equipment like autonomous robots that move products on the factory floor and move heavy materials at just the right time.
Industrial smart devices use sensors to collect data to make autonomous decisions while simultaneously communicating with a more extensive system. Automated communication enables independent synchronization across an entire smart factory.
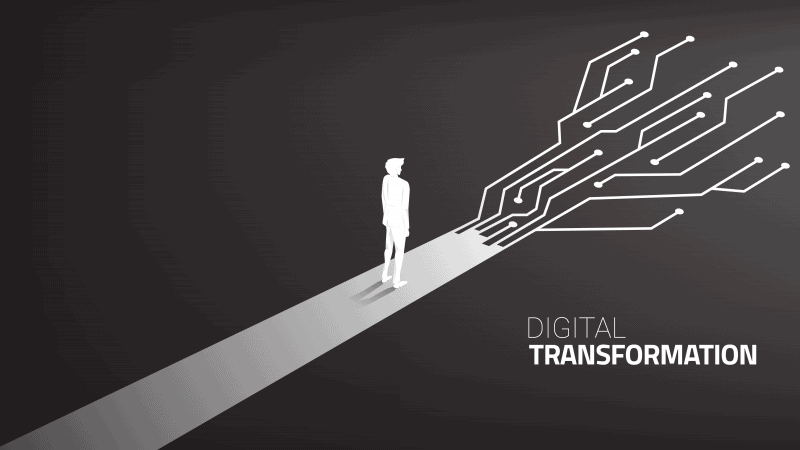
Digital Transformation
To realize Industry 4.0, companies must go through a digital transformation. Digital transformation is a strategic initiative to move an organization from analog to digital methods or from digital processes to an automated systems strategy.
Digital transformation is typically an enterprise-wide initiative focused on cutting costs and increasing profit margins through digitalization. Industry 4.0 is part of the digital transformation processes for the engineering, procurement, and manufacturing divisions of a company.
IIoT
The new digital era is driven by a web of connected devices or the “internet of things” (IoT). A Smart fridge, The Apple Watch, Nest’s home thermostat, and Amazon Alexa are all examples of independently connected devices that communicate data to other devices and people over the internet. The culmination of all “smart devices” makes up the Internet of Things.
Likewise, the Industrial Internet of Things or IIoT consists of millions of connected devices for manufacturing, packaging, warehousing, and shipping facilities. IIoT brings manufacturing machines to life by adding sensors to critical components that share data. This data feeds into business systems and is analyzed to determine production time, potential delays, and maintenance schedules.
Industrial Big Data
All the connected IIoT devices and other industrial data feed unfathomable amounts of data to various business systems. The aggregate of all of this collected industrial information is known as Industrial Big Data. Storage and networks are currently being deployed by manufacturing companies worldwide to handle the increase in data bandwidth.
Larger data storage capacity and networks with higher bandwidth capabilities are only one of many challenges Industrial Big Data has introduced. Another major challenge is the ability to analyze all of the newly created information and translate the information to the right machines and people at the right time.
Data Intelligence
Industrial Big Data requires companies to analyze data and turn it into usable insights to drive business decisions; this is data intelligence. The significant change Industry 4.0 brings to data intelligence is the sheer volume of data that sensors collect across various connected systems. The information is being communicated at a rate too fast for people to analyze it on their own.
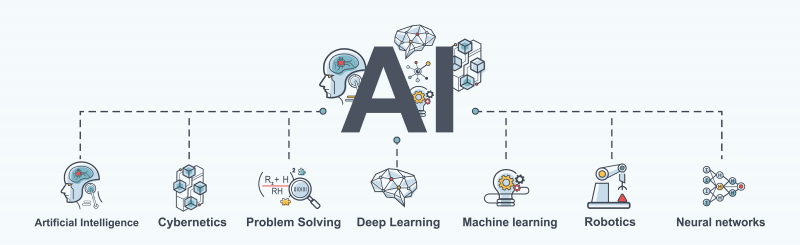
Artificial Intelligence (AI) & Machine Learning
The future of Industry 4.0 requires automated analysis of data and communication using artificial intelligence and machine learning. Artificial intelligence, or AI, simulates human thinking and decision-making using advanced algorithms. Machine learning uses past data to give more efficient and accurate outputs or complete specific tasks based on past data.
Machine-to-machine communication (M2M) is paramount for Industry 4.0. Machine-to-machine communication enables manufacturing machines to send data to other devices and software applications that use machine learning and AI to complete tasks.
For example, a machine on the manufacturing floor with a built-in thermometer can signal when the machine’s risk of overheating is high. The alert can trigger an algorithm that slows down all the proceeding machines in the production line, allowing the machine to cool down.
Simultaneously, the algorithm can activate a cooling system with the exact temperature to restore optimal production. The system can also send a push notification to the facility manager’s phone, showing her how the production slowdown will affect product output.
Finally, the system sends updated production data to other business systems updating supply chain, sales, and the executive team about delays and exact dollar losses from the slow down.
When machine-to-machine communication is operational, it enables a digital thread of communication to pass to the right people and devices at the right time.
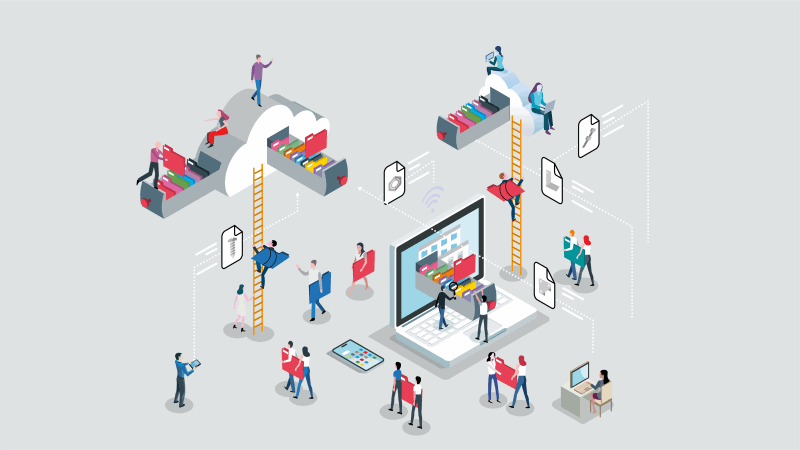
Digital Thread
The digital thread describes a digital line of communication that breaks the barriers of traditionally siloed departments. Digital thread initiatives aim to push decision-making power to the lowest level possible by giving relevant information to the right person, in the right department, at the right time.
Digital thread pricing example
The digital thread looks, in practice, like someone in procurement adding pricing information to a purchased part in their ERP system. The system automatically updates the company’s PLM system. Now engineers can see pricing when searching for a component within their connected CAD program, enabling better decision-making.
Digital thread engineering change example
Another example of the digital thread is a customer calling a feedback number on the back of a shampoo bottle to complain about how the cap doesn’t stay closed. The customer service rep, on the other end, inputs the information into their feedback systems. Machine learning technology looks for similar feedback from other customers. Once customer feedback on the issue reaches a threshold, an engineering manager gets a notification to their smartphone detailing the malfunction’s nature.
The engineering manager puts his team to work fixing the issue. Once the new product revision is live, the updated version of the product passes directly to manufacturing software, and the change goes into production on the next batch of shampoo lids.
Machine learning and artificial intelligence can analyze data from many departments and automatically push the data throughout the organization in a format that can be read by the appropriate machine or person receiving it. Moreover, this whole process happens in real-time.
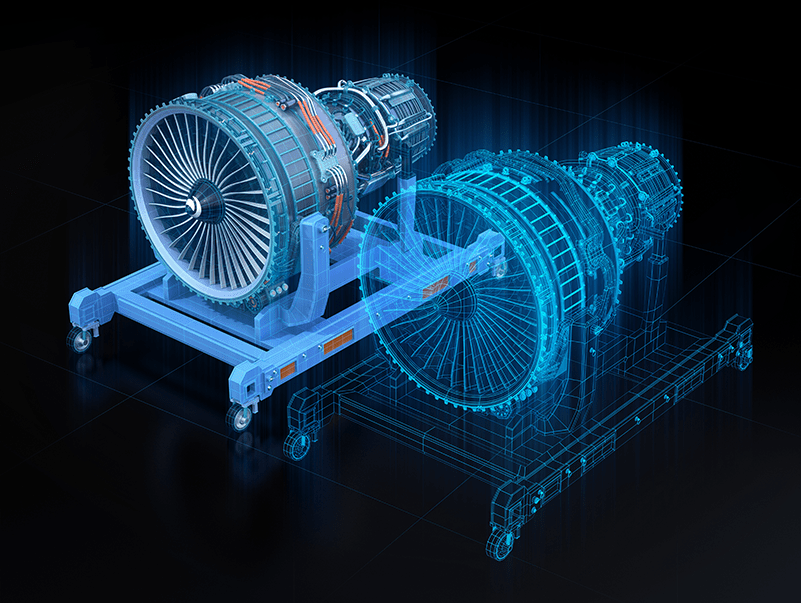
Digital Twin Technology
Digital twins are digital representations of physical or potential physical objects, mainly used for digital testing, digital simulation, predictive maintenance, and analysis.
Examples of digital twin technology:
Digital Twin for Engineering Design, Simulation, and Testing
Digital Twin technology for Industry 4.0 can increase speed to market by enabling design engineers and industrial designers to virtually test and simulate products while still in the design phase.
For example, a design engineering team at a consumer goods manufacturing company can test and simulate bottle design and liquid flow for a shampoo bottle. This type of digital twin testing enables the team to see how a shampoo bottle will work virtually.
Using digital twin technology, engineers can do virtual tests instantly before real-world testing, shaving weeks or even months from the process. Then, using data from these tests, engineers can make changes immediately and test the product again, getting near-instantaneous results from design changes.
One executive from a fortune 100 manufacturer said that digital twin virtual testing for design cut their time to market for new product SKUs from three to 6 months to less than six weeks. In addition, digital twins have helped them address new consumer demands within weeks instead of months or years.
It also helps engineers test design changes from customer feedback, address design flaws, and release a final manufacturing revision within weeks. They can address customer concerns and fix faulty products much faster using digital twin technology. Digital twins enable actionable customer service, increasing customer retention, reducing recalls, and making for much happier customers.
Leveraging digital twin technology for design simulation is a competitive advantage for large enterprises, making it harder for smaller companies to disrupt them.
Digital Twin for Maintenance and Performance Analysis
GE Aviation is leading the charge in digital twin technology for maintenance and performance analysis. They are creating digital replicas (digital twins) for each new GE engine that goes into service. These digital twins collect and analyze real-world data.
Smart sensors collect data within each engine. Flight data like flight duration, weather, and altitude are also collected. One intercontinental flight has the potential to amass terabytes of data. All of that data is analyzed using AI and machine learning. A computer system uses the data to predict maintenance schedules, end of life for engine parts, and analytics for future design improvements.
Digital twin technology saves millions of dollars for GE Aviation customers by reducing downtime from previously unpredictable engine maintenance. It also increases airplane safety by determining potential fail points and mitigating them before a disaster occurs.
Manufacturing companies can harness the GE Aviation digital twin example for Industry 4.0 by creating digital twins for IIoT enabled manufacturing machines. Embedded sensors on the devices can communicate usage and wear and tear data to a control system. In addition, AI-driven data intelligence will analyze the digital twin data and production capacity, room temperature, scheduled maintenance, and downtime cost data for predictive maintenance.
Component Digital Twins
Component digital twins is a new use case of digital twin technology. Forward-thinking component manufacturers like Festo and Balluff deliver Industry 4.0 ready CAD models for the products they sell. Engineers can configure parts available from these companies and download them in a format that keeps the data’s fidelity. The component digital twins enable smart digital technologies downstream from engineering.
We discuss this topic in-depth in the ‘Data is the keystone of Industry 4.0’ section of this article.
Learn how you can offer CAD that is ready for the future. Engineers need data for IIoT, programming, and factory automation. They require more data from suppliers. Set your components apart by giving engineers the CAD data they need.
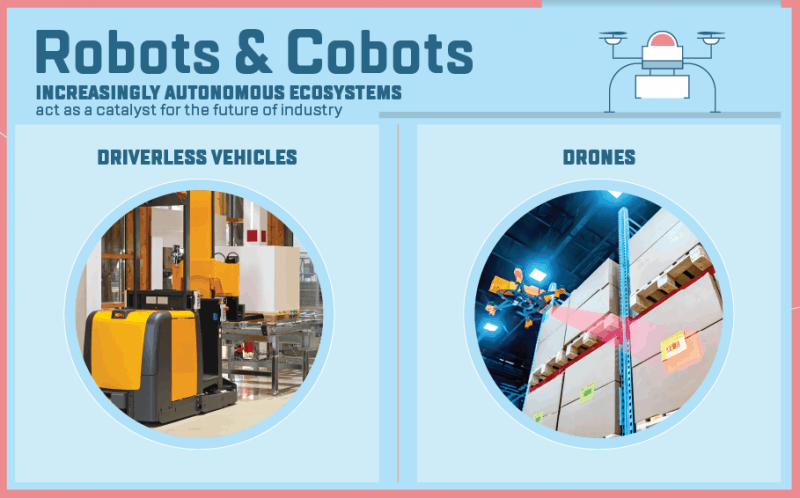
Autonomous Robots and Cobots
Autonomous robots are robots that work independently of humans or pre-programmed routes and actions.
Autonomous robots aren’t limited to the simple home vacuum anymore. Because of advancements in high-precision visual sensors, autonomous robots are debuting in agriculture, food products, and mass production manufacturing facilities. Autonomous robots use a mix of AI and machine learning capabilities to accomplish necessary tasks and facilitate production and logistics more efficiently than humans.
Robotic machines now perform tasks that formerly required human intelligence. For example, autonomous mobile robots now operate in connection with hundreds or thousands of other robots. Using industrial big data, robots can function separately without getting in the way of other robots or machines.
Another essential function of robotics in manufacturing is the cobot.
Cobots are robotic partners that work alongside humans to augment their abilities and production output. This partnership enables a person to work in conjunction with automated equipment to multiply their work.
Many tasks in manufacturing require human cognitive ability and outside-the-box thinking. Cobots enable people to focus on what humans do best while saving time on simple multi-tasking that can be easily automated.
Factory Automation
The end goal of Industry 4.0 is to automate factories and manufacturing processes. “Lights out manufacturing” is a term used to describe a fully automated factory with no human involvement, making turning the lights on optional.
We are still a long way from having fully automated factories. However, humans play an essential role in quality control, critical thinking, and social interaction. AI is not nearly advanced enough to completely eliminate the need for humans in manufacturing anytime soon.
However, most manufacturing tasks can and will be automated by advanced robots over the next few decades. Automation will make technologies cheaper and more widely available for markets that previously could not access the essential benefits of mass production.
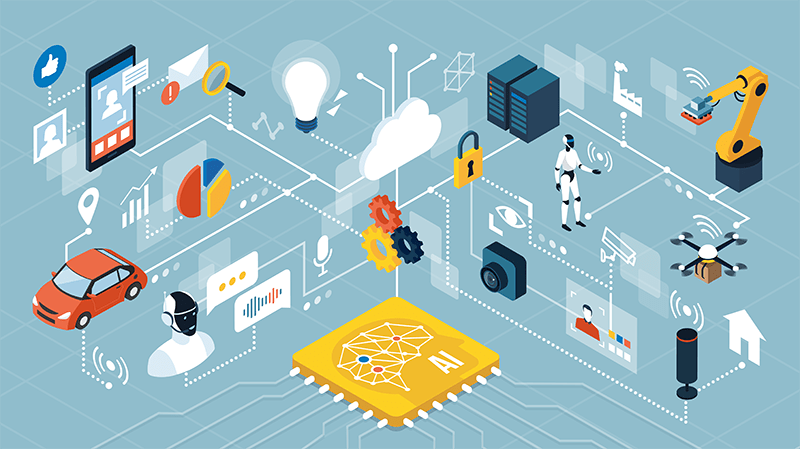
Predictive Maintenance
Manufacturing equipment breaks. It’s a reality of doing business. Companies can lose significant money when a machine goes down, especially when it is down for an extended time. Equipment maintenance can be expensive, but having an assembly line down for days, or even for a few hours, can cost millions of dollars in lost production.
One promising aspect of Industry 4.0 is the ability to mitigate the risks involved with maintenance. For example, machines equipped with smart parts can send a signal when they are not functioning at the optimum level. Alternatively, engineers can create a digital twin of the machinery to simulate when different components need replacement and where the financial tipping point is for performing the maintenance.
AI and machine learning technology can send alerts to a maintenance manager warning of potential equipment failure before it happens.
Predictive maintenance enables real-time maintenance reporting to facilitate the purchase of parts and scheduling downtime for machines limiting costs from lost production.
Additive Manufacturing & 3D Printing
We can’t move on from Industry 4.0 technology without addressing additive manufacturing, also known as 3D printing. There is a variety of techniques and materials for additive manufacturing. For instance, metals and plastics are two primary mediums for 3D printing.
Instead of stamping, punching, casting, and machining or casting and injecting plastics, additive manufacturing layers on materials one microlayer at a time to create a 3D product or component. 3D printing enables product designs that were impossible to create with the old style of manufacturing.
Additive manufacturing is a fantastic catalyst for automation because it doesn’t require retooling to change designs. If an engineer, or even AI, makes changes to the design within an additive manufacturing program, the changes can instantly affect production.
Digital Procurement
Many procurement processes are still managed in excel or similar low-tech methods. Digital procurement is the process of adopting strategic sourcing systems that enable supply chain predictions, AI-driven cost reduction, and automated purchasing of parts.
Digital procurement will be a vital part of successfully implementing Industry 4.0, although the technologies are still being rolled out.
IT Infrastructure for Industry 4.0
New IT infrastructure and networks are how IIoT devices can communicate. Without updating IT infrastructure, Industry 4.0 is only a pipedream.
Here are a few essential Industry 4.0 IT terms:
Cloud Computing
At this point, most of us are familiar with “the cloud” from cloud-based software like Google Cloud, iCloud, Dropbox, and Microsoft 365. More and more of the applications we use daily run in the cloud. The physical equipment that powers cloud environments is typically spread across multiple physical IT facilities worldwide.
Cloud computing uses cloud technology not only for storage but also for high-bandwidth computer processing. This infrastructure means IIoT equipment can send data to a cloud computing system where AI can perform automated data intelligence in real-time and store the information in a central cloud system.
Cloud computing decentralizes a company’s computing systems, providing better security, up-to-date systems, and data storage redundancy. It also merges multiple data streams in one location, closing the digital thread and communication loops.
5G and Industry 4.0 Networks
Real-time cloud computing and storage of data require more robust integrated networks than existed in the past. Facilities need new hardware to enable data-intensive network capabilities. Some of these IT technologies are only now emerging on the market.
5G technology will significantly expand the capacity of wireless networks. With peak speeds up to 20Gbs, 5G opens the door to new technologies that were impossible to implement in the past. Compare those speeds to 4G wireless, which has a real-world peak speed of 100Mbs.
5G and other emerging network technologies are at a point where IIoT is becoming a reality. However, without a robust networking strategy, it is impossible to implement Industry 4.0 technologies.
Internal Networks and Enterprise Networks
Internal networking is the connection of network devices that communicate together in a closed communication loop. A closed communication loop is when machines rely on other machines’ data to operate independently from external systems.
Think of a group of manufacturing machines that depend on each other to operate. The communication is only between these machines, which is a “closed-loop” of communication. One apparatus may handle material, another one cuts the materials, and finally, the last device punches out the widget. These devices are automation-ready since they communicate together on a network.
Enterprise networking is when network devices communicate with systems outside of their direct closed loop of communication. For instance, the computer program that runs the material handling, cutting, and punching machines from the previous example may communicate with other systems within the manufacturing facility. It may also communicate back and forth with systems outside the manufacturing facility.
In this scenario, the manufacturing system can run at the optimum speed based on other manufacturing machines’ production capacity. The system can also send production information to a central hub network, managing material inventory, and purchasing. It can also send data to other systems that analyze potential production delays and communicate to a management team the cost of production slowdowns. This process is an example of an enterprise network.
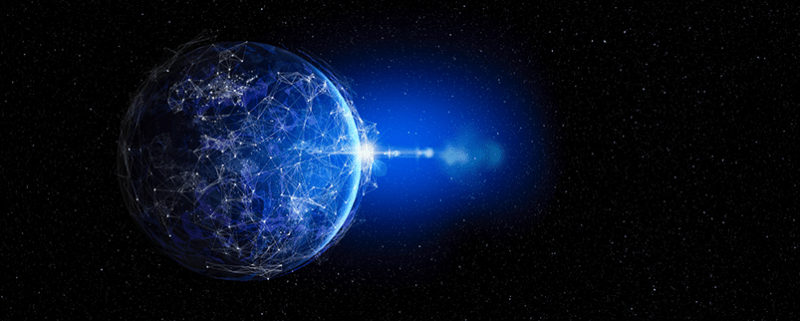
Edge Computing
The amount of data communicated by sensors within large-scale facilities often exceeds the current networking capacity. Edge computing allows internal networking data processing to happen at a facility. Thus, only important information is passed through enterprise networking for processing in the cloud and sent to a central system, reducing the network’s bandwidth burden.
Cybersecurity
With all tech advances, new challenges arise. For example, a significant concern with implementing automated systems and data flow is cyber crimes. Ransomware takes on a whole new meaning when the perpetrators take over an entire factory remotely and hold the factory for ransom.
The cybersecurity industry is booming right now, and for a good reason. Companies looking to deploy IIoT and Industry 4.0 tech must consider cybercrime risks and mitigate them with a cybersecurity strategy.
Understanding the risks of fully automated systems enables companies to develop a strong cybersecurity plan.
IT Technology is the Means, Not the End Goal
If Industry 4.0 was a garden, IT Infrastructure is like a hose, and data is like water. The goal is plant growth. To grow, a garden requires water. Likewise, for water to get to the garden necessitates the use of a hose.
The goals of industry 4.0 are to increase efficiency, cut costs, and improve customers’ lives. To enable automated technologies, we must have high-fidelity data. IT infrastructure is vital, but it is the means of moving and storing data.
There has been much emphasis on IT environments and equipment, but we can’t lose sight of why we are implementing new systems. Reliable network capabilities are valuable if they facilitate the collection of relevant and accurate data.
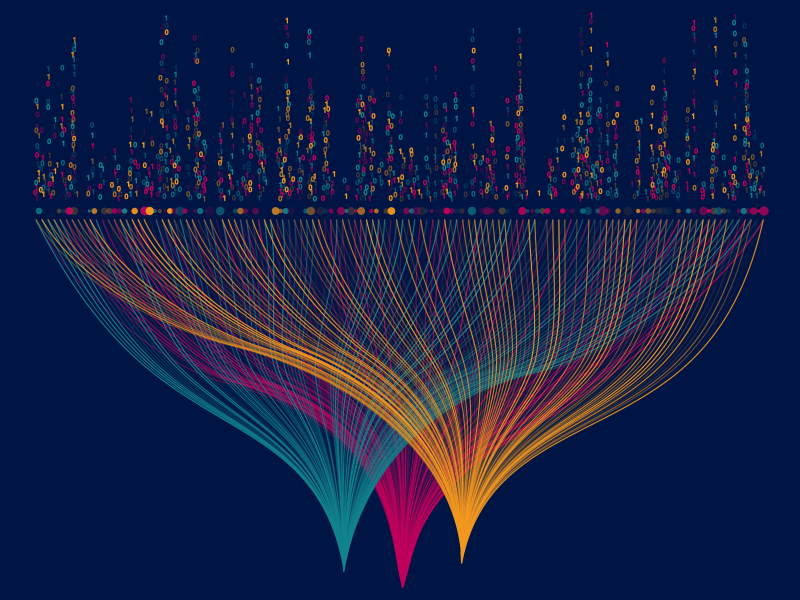
Data is the Keystone of Industry 4.0
Companies that want to leverage industry 4.0 must consider data flow both within and outside the organization. The establishment of a digital thread of information throughout every department in a company is paramount. In addition, it is imperative to ensure data fidelity in departments that introduce new data like engineering, procurement, and manufacturing.
If just one crucial portion of data is missing, it breaks the digital thread, and the flow of data stops. Creating a digital thread of information is commonly implemented for the internal data a company generates, but what about supplier and partner data?
The digital thread MUST extend beyond your internal operations to encompass supplier and partner data as well.
Engineering data is the perfect example of why high-fidelity information is vital for internal and supplier data.
High-Fidelity CAD Data: The Missing Link
Industry 4.0 is not possible until the correct data is present within CAD models in a format that can be read into many systems and is usable downstream from engineering. Engineering initiatives like model-based enterprise are the first steps to ensuring this happens, but there is still a long way to go.
Model-Based Enterprise
Model-Based enterprise uses CAD data as the “single source of truth.” Engineers must add all information relevant to downstream processes to CAD models, such as manufacturing data (MBD), supply chain information, pricing, and even maintenance and end-of-life data.
Implementing a model-based enterprise initiative is an essential aspect of realizing Industry 4.0. For example, suppose engineers aren’t entering all relevant data into the correct business systems at the source. In that case, no network speeds, machine learning, or artificial intelligence can bridge the low-quality data gap.
Here are some examples of critical internal data:
Model-Based Definition (MBD)
In the past, engineers had to create a 3D CAD model first, then originate a 2D drawing with the exact measurements and manufacturing requirements. The manufacturing team would then translate the 2D drawing to a 3D model read by a CAM program or other manufacturing software. Unfortunately, the 3D CAD model wasn’t compatible with manufacturing software programs. Therefore, the extra steps of manually translating two and from 2D CAD was a requirement.
Because of these inefficiencies, some companies have introduced model-based definitions to their 3D CAD drawings. The idea of model-based definition is to provide all the product manufacturing information (PMI), like part dimensions, special instructions for CAM software, and other manufacturing needs, directly within the CAD format that can be read into manufacturing software. Thus, model-based definition cuts human costs, decreases lead time for manufacturing parts, and eliminates costly human errors from the process.
Internally designed MBD CAD Models should include PMI
Product manufacturing information (PMI) is the data needed for manufacturing machines to produce a part. PMI is information like:
- Geometric dimensions and tolerances (GD&T)
- Material and finishes
- Manufacturing notes and annotations
- Critical design intent
Manufacturing companies use PMI to program machines and give guidance and specifications for the final component.
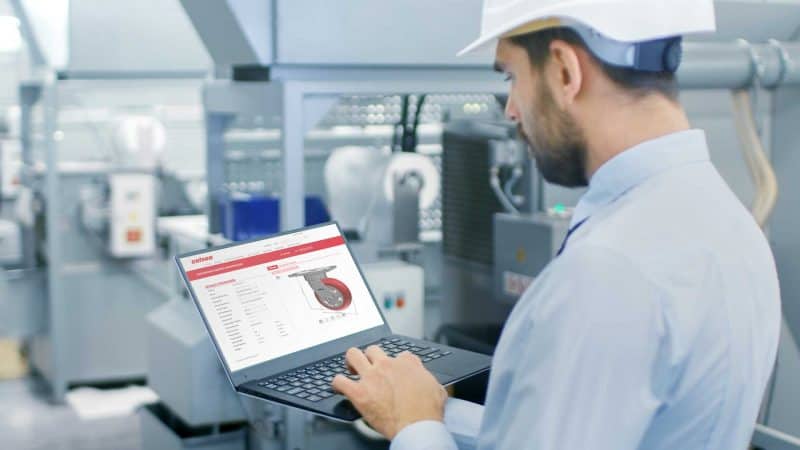
Design Intent
Design Intent is the why and how behind an engineer’s purpose in creating a component. It allows engineers to change 3D models while still maintaining the initial objective of the part.
Data that shows design Intent are attributes like:
- Annotations
- The feature tree
- History
- Software specific parametric programming
Much design intent is lost when translating from a native CAD format to a neutral CAD format. This is why it is vital to create a strategy whereby engineers use native CAD formats, or where CAD is translated properly by CAD translation software, as much as possible. Neutral CAD formats like STEP were created for long-term archival and are still best suited for that purpose because they don’t preserve vital design intent information.
Design Intent data enables humans and machines to understand the why and how behind the design of a 3D CAD model. It provides critical data for other engineers, manufacturing, procurement professionals and powers AI and machine learning.
Motion Study & Kinematic Information
Many physical components and assemblies have moving parts. To enable simulation, testing, and digital twin capabilities, 3D CAD models must contain the proper metadata.
The parts must be mated and constrained, have the full range of motion and kinematic data. Using this data, humans and machines can simulate how a CAD model will function within an assembly.
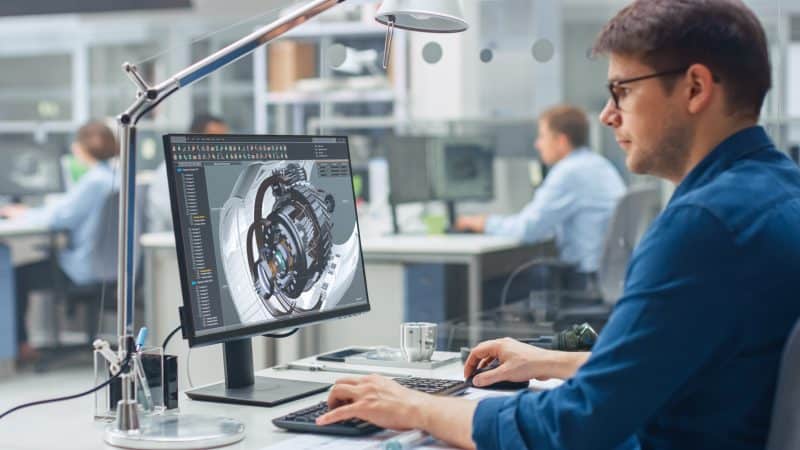
Testing and Simulation Data
After engineers run testing and simulation programs, tolerances, point of failure information, and end-of-life data must be embedded into the metadata of each CAD model. In addition, test results and simulation data are vital for Industry 4.0 predictive maintenance.
This type of projected function data is utilized by automation software that collects data in real-time from IIoT devices and compares it to the tests. The automation software can then make new projections based on analyzing the predicted function and actual function of the machines and components.
Pricing and Availability
To increase efficiency and automate purchasing, engineering and procurement departments require pricing and availability information within CAD models’ metadata. Systems like CADENAS PARTsolutions parts management software work as a catalyst for engineers and procurement professionals to access vital product information.
Engineers can make better decisions if they have pricing and availability on parts that already exist in their PLM, PDM, and ERP systems. In addition, this type of information empowers engineers to reuse CAD models and CAD data from previous projects.
Procurement professionals can find and ultimately automate purchasing decisions based on the geometry of a part using 3D shape search software to find similar CAD models in their systems.
Parametric Information
All parametric information needs to be accessible in different stages of the product life cycle. Now more than ever, it’s vital for people and machines in manufacturing, procurement, and sales to have access to 3D models. Furthermore, these models must maintain accuracy even after being exported from the original native CAD format to a neutral CAD format or through structured data export like QIF.
Data capture and communication are the first steps in the digital thread for manufacturing, ultimately leading to automated repeat purchases and leveraging machine learning to get better pricing from suppliers.
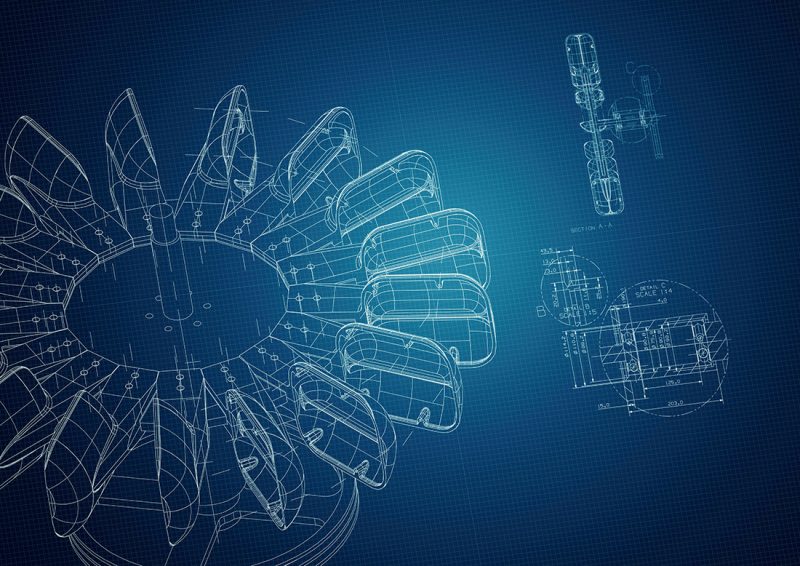
Require Component Digital Twins from Suppliers
One challenge to implementing a fully model-based enterprise and achieving Industry 4.0 is the lack of quality supplier data, especially supplier CAD data. To understand why supplier CAD data is of such poor quality, you first must understand how engineers are getting supplier data.
Engineers typically access supplier data in one of three ways:
- Through a physical or digital supplier spec sheet
- Requesting a CAD model from a supplier
- Downloading a neutral CAD file from the internet
These methods present issues both with the lack of efficiency for engineers and with the low data quality.
Spec Sheets
When using a supplier spec sheet, a design engineer must create a CAD model representing the supplier’s data.
The first issue with this method is the margin for human error when translating the information from a spec sheet to a 3D CAD model. Engineers typically only embed the metadata they need for their specific job. Data required for downstream processes often won’t make it into the model.
Having engineers create 3D models from parts that already exist is a colossal waste of time. Engineers admit to spending 25% of their time searching for and recreating supplier parts.
That’s a lot of time!
Requesting CAD
If a part is complicated or requires customization, engineers will request a CAD model from the supplier. It typically takes three to fourteen days for the engineer to receive the CAD model. The model is typically a neutral CAD format, which doesn’t have essential metadata required for Industry 4.0 initiatives.
Downloading a neutral CAD file from the internet
The final way engineers gain access to supplier data is by downloading a CAD model from the supplier’s website or a third-party site. Unfortunately, CAD from supplier websites is often delivered in a neutral CAD format, lacking quality data, or only offered in one native CAD format.
All these options slow down the engineering process, weaken component manufacturers’ ability to sell parts, and are full of low-quality CAD data.
That’s where component digital twins come into play.
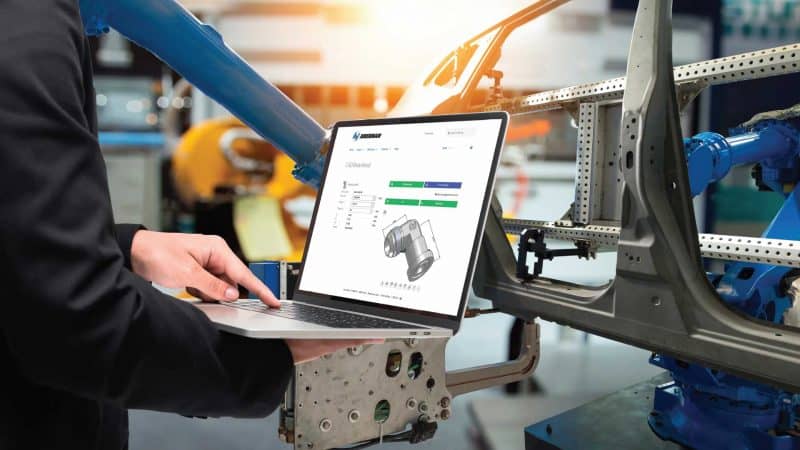
What are component digital twins?
Component digital twins are high-fidelity native CAD models that are equipped for industry 4.0. Native CAD formats are the power behind component digital twins. Native CAD formats preserve design intent and contain critical data.
Supplier Component Digital Twins
Typically, component manufacturers provide a neutral CAD file for engineers to spec into their designs. That worked great in the past when engineers needed less metadata embedded in the CAD model.
Today’s engineers need component digital twins. To meet crucial Industry 4.0 requirements, component manufacturers must provide CAD models in a native CAD format. By offering native CAD formats to engineers, the manufacturer’s CAD files match any CAD software their customers use, preserving vital metadata and design intent.
Native CAD files can store data necessary for departments downstream from engineering when compared to neutral CAD formats.
You can learn about the differences between Native CAD formats and neutral CAD formats in this eBook.
Learn how you can offer CAD that is ready for the future. Engineers need data for IIoT, programming, and factory automation. They require more data from suppliers. Set your components apart by giving engineers the CAD data they need.
Metadata for Industry 4.0
CAD metadata is data embedded within a CAD model that provides supporting information, increasing data fidelity. Here are a few examples of CAD metadata for enabling Industry 4.0 functionality:
- End of life information
- Obsolescence
- IIoT tags
- Materials
- Finish
- Tolerances
- Battery capacity and longevity
- wattage
- Speeds, horsepower, max RPMs
- Full range of motion data
- Unique manufacturer part number
The metadata needed varies by component type.
When making cost projections for a project, it is critical to factor in the cost of collecting and embedding this metadata if your suppliers don’t provide it. When engineers must manually collect and embed the required CAD metadata for Industry 4.0, supplier parts add high costs throughout the product life cycle. Having to collect this information causes bottlenecks and breaks the digital thread.
When suppliers provide Industry 4.0 enabled component digital twins, they save engineers much time and ensure data quality for their customers.
In some cases, having high-fidelity metadata certified by the manufacturer is worth more than the component itself, considering the cost of finding and adding the data after the fact.
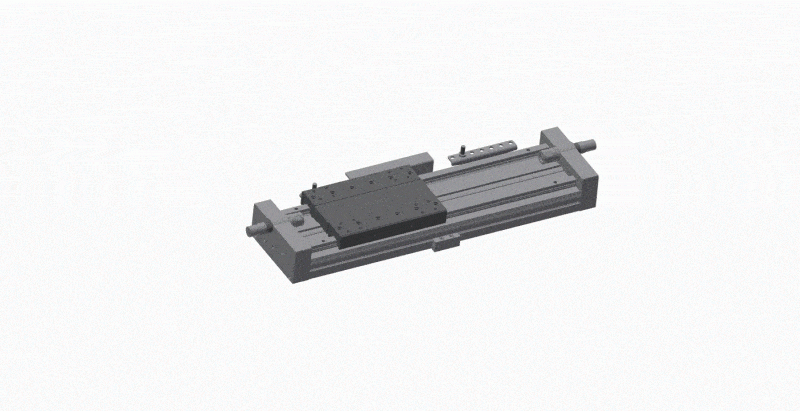
Why Component Digital Twins are Good for Manufacturers
Component digital twins give manufacturers a competitive advantage now and future proof their CAD catalog investment as more companies require Industry 4.0 metadata from suppliers.
The second reason is that component digital twins are more likely to be purchased by procurement professionals and digital procurement software. Purchasing friction is reduced because component digital twins include the unique manufacturer part number within the CAD model.
When engineers spec components into a design, a company’s sourcing team must purchase the part before it goes into production, if the manufacturer’s part number is embedded in the CAD model’s metadata, the part number can be transferred directly to the BOM (bill of materials).
If a manufacturer’s part number is on the BOM, it makes it easy as pie for procurement to purchase that exact part. If they have to search for a part number, they may look up competitive parts.
Component digital twins also enable automated procurement software to purchase the manufacturer’s part, increasing sales for manufacturers.
Industry 4.0 for procurement necessitates that suppliers provide component digital twins so that the metadata is available to use automation purchase parts for the initial production and automatic delivery of parts for maintenance.
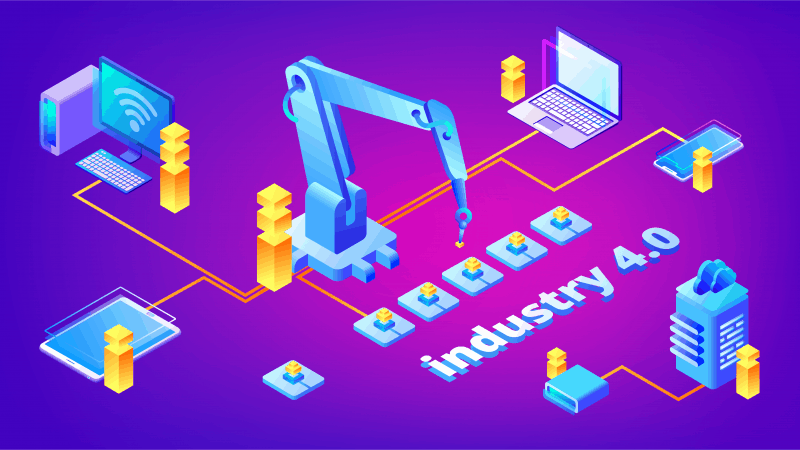
Is Your Data Ready for Industry 4.0?
The new Industrial Revolution is underway. The factories of the future will be functioning with few humans on the factory floor. Productivity and safety will improve. And the market will get better, more customized products, in less time, at a lower cost.
Is your company ready for Industry 4.0? Have you considered how your current strategy can be disrupted by factory automation and the market’s demands?
For the component manufacturers out there, has your team considered how the demand for Industry 4.0 enabled CAD models and component digital twins will affect your business? Do you currently have a strategy for delivering the CAD data your customers will require in the future?
These are central questions to think through for businesses looking to survive the new Industrial Revolution. Forward-thinking companies have already begun their digital transformation and are prepped for a significant competitive advantage as the missing pieces of Industry 4.0 come together.
Additional Industry 4.0 Sources:
https://iiot-world.com/industrial-iot/connected-industry/nine-challenges-of-industry-4-0/
https://www.challenge.org/industry-4-0-3-3/challenges/
https://eacpds.com/what-is-a-digital-thread/
https://www.linkedin.com/pulse/top-quotes-industry-40-abbey-heffer/
https://ied.eu/project-updates/the-4-industrial-revolutions/
https://www.britannica.com/event/Industrial-Revolution
https://automationalley.com/Blog/2019/August-2019/7-Inspirational-Quotes-on-Industry-4-0.aspx